How do we get autonomous vehicles to be more ‘human’? Perceptive Automata, a Somerville, Massachusetts based startup, has developed a solution that can help autonomous vehicles intuit human behaviour, ensuring safer deployment. It all started with an experiment.
The Experiment
In early 2016, Sam Anthony, CTO and Co-founder of Perceptive Automata, placed a GoPro camera at an intersection to answer one simple question – “while driving, how often do people look at another person and attempt to understand and react to what that person is thinking?”.
Upon analyzing the videos, he realized people are constantly making subconscious judgements about others actions. In a 30-second segment, he found over 50 instances of such subtle communications. For example, a scooter at the intersection wants to drive through but is waiting to see if the oncoming cars will let him pass. Another vehicle comes along and wants to turn. The scooter moves back in response to seeing and is signalling that he recognizes the other person wants to turn and needs space.
Machines lack this ability. When they detect something in the surroundings, they slow down or come to a complete stop. This could be problematic as it may not be the right response. The inability to properly understand its surroundings has resulted in accidents. A classic example of this is Google’s first crash back in 2016.
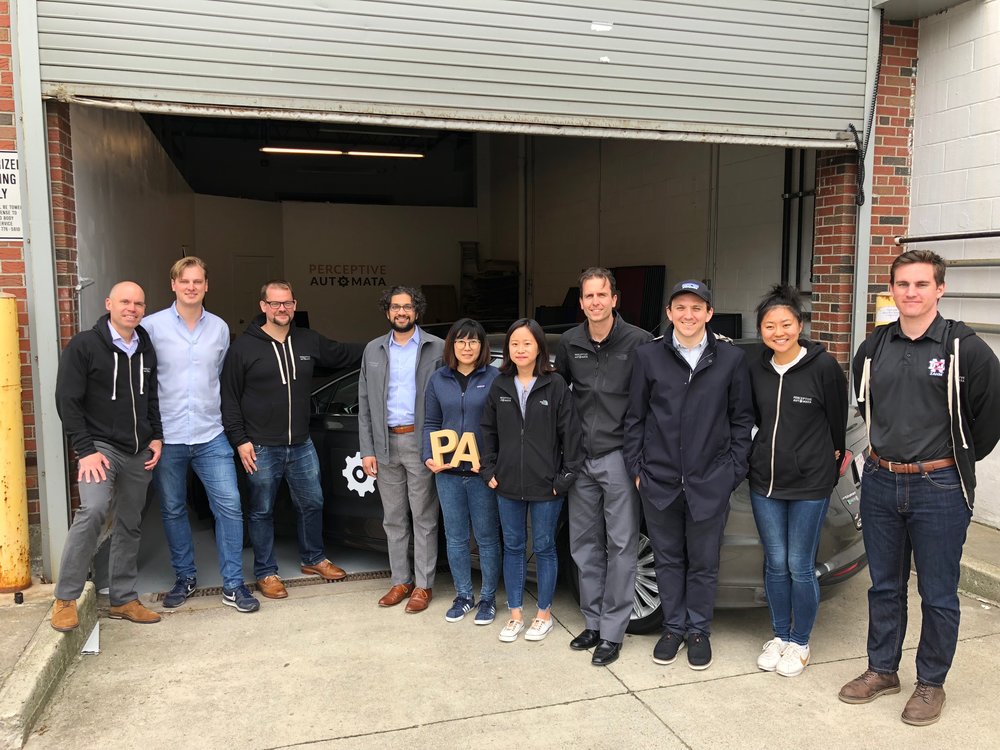
Team Perceptive Automata (PC: Perceptive Automata)
Where’s the Autonomous Vehicle?
There are six levels of driving automation ranging from no driving automation (level 0) to full driving automation (level 5) as defined by SAE International. Our understanding of autonomous cars from pop culture falls in the level 4 (high driving automation) and level 5 categories.
Growing at a CAGR of 39.5%, the global autonomous vehicles market is poised to reach $557Bn by 2026. But things might get delayed. The recent Gartner Hype Cycle places level 4 in the early stages of the ‘trough of disillusionment’ and level 5 at half way through the ‘innovation trigger’. While experts may have diverging estimates ranging from a few years to a few decades on when autonomous vehicles will hit the road, it is safe to say it won’t be anytime soon.
Auto tech continues to be a highly anticipated area with autonomous vehicles startups getting most of the investment. Thus far in 2018, we’ve already seen over 116 deals with a total of $5Bn in funding. Money pours into startups that are helping solve the many challenges on the road to safe deployment. There are many challenges such as mapping, trajectory optimization, scene perception that remain to be solved before we see safe deployment of autonomous vehicles. Higher-level planning decisions is also a challenge and requires modelling human intuition.
Machines vs Humans
In a paper published by University of Michigan Transportation Research Institute (UMTRI), Brandon Schoettle finds that machines are well suited to tasks that require a quick reaction time or consistency or processing lots of information from different sources. However, when it comes to reasoning and perception, humans have an advantage. It is an intense process for autonomous vehicle to reach this level.
There is a fundamental issue with the traditional methods of training machine learning models. The traditional paradigm is pretty straightforward in terms of input and output. There are images based on which the system is trained and the ‘goodness’ of the model is based on its performance on a sample taken from the data set and is measured against a benchmark. How do you know if the model has truly learned the principles that guide decisions or it is simply matching using memory?
While describing the problem with the traditional models in a paper on visual recognition, the co-founders touch upon the classic Chinese Room problem proposed by the philosopher John Searle, where a man who doesn’t speak Chinese is locked up in a box with a book/set of instructions. His task is to generate Chinese characters in response to a message slipped in (input) while relying on the book. The person receiving the final result (output) may think that the person inside the box knows Chinese, but this isn’t the case. This has real life consequences as when the system is put on road, it is important that the system truly understands its surroundings.
As one of the major deployment areas for autonomous vehicles is densely crowded urban areas, it is important for autonomous vehicles to be in sync with humans. As seen from Sam’s experience, we find instances of non-verbal communication between the drivers and pedestrians or other drivers which are intuitive and processed at a subconscious level. Is that person going to cross the road or will the person wait? Such problems require a more profound understanding of human behaviour rather than simply identifying objects in the surroundings.
The Perceptive Automata Solution
Perceptive Automata’s solution is based on visual psychophysics, a branch of psychology that deals with the relationship between stimuli and behavioural responses, as a paradigm to develop computer vision. This method helps quantify, measure and incorporate behavioural aspects into these models.
As opposed to the traditional model, this approach relies on the use of ‘perturbation’ which distorts the image using blurs and rotations and then requires the model to identify the image. In another paper, the co-founders apply a similar approach to facial recognition while making use of facial expressions and distortions such as contrasts to check accuracy. By comparing the model’s results with human results, the papers demonstrate areas where models are truly ‘super human’ and where human are better.
Perceptive imagines self-driving cars which can predict behavior
Based on these models (and more), Perceptive Automata’s highly sophisticated algorithms have a richer understanding of their surroundings. Below are a few graphics that help illustrate their approach. The green eye signals that the person is aware of the vehicle’s presence or has seen the vehicle while the redness of the bar indicates the intent to move. When the ‘eye’ is covered by the red circle and line, it indicates that the person isn’t aware while the white bar indicates no intent to move. These features help the vehicle understand and embed itself in its environment more easily.
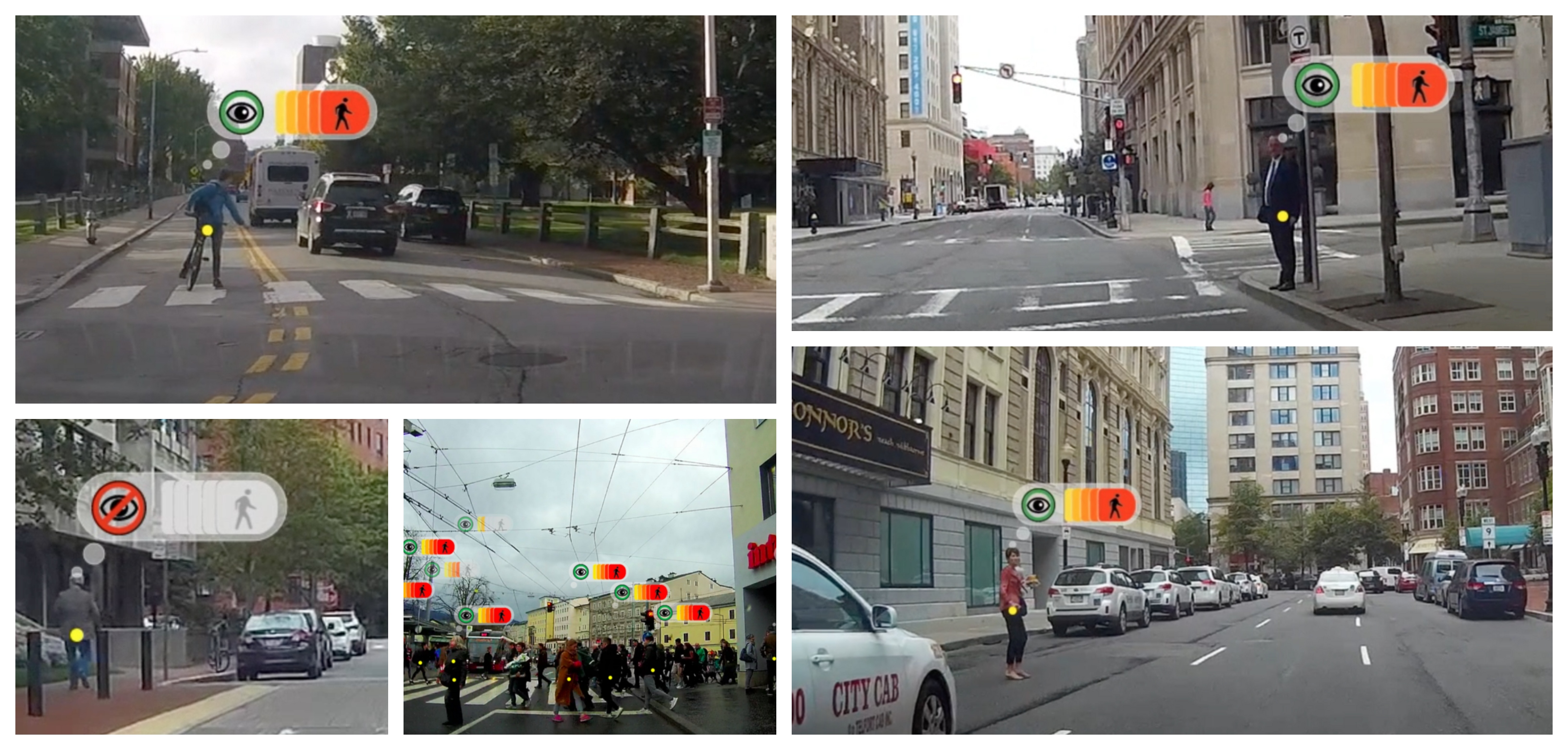
Algorithm visualizations (PC: Perceptive Automata)
The Perceptive Future
The four-year-old startup was co-founded by Sid Misra (CEO), Dr. Sam Anthony (CTO), Avery Faller (Senior Machine Learning Engineer), Dr. David Cox and Dr. Walter Scheirer (advisors continuing their academic work). The team is comprised of Harvard, MIT, and Stanford neuroscientists and an AI expert. The idea was conceived while the co-founders were at Harvard. Perceptive Automata was in stealth mode for a very long time, until July this year when the team unveiled their work. They recently raised $16M in series A funding led by Jazz Venture Partners with participation from Hyundai Motor Company, Toyota AI Ventures and existing investors First Round Capital and Slow Ventures. Having raised $3 M from First Round Capital and Slow Ventures last year and received grants to the tune of $1 M, Perceptive Automata has raised $20 M thus far. Their immediate focus now is to grow the product development and customer implementation teams while also bringing on more top engineers to further refine the software.
While there are numerous players spread across the landscape focusing on solving different challenges, the broader market can be looked at from the perspective of full stack developers (who work on everything from the idea to the final product) and ‘others’. Perceptive Automata competes with in-house teams at Waymo, Uber and other full stack developers while at the same time, being a potential solution provider to these firms and the ‘others’. Humanizing Autonomy, a London based company working on developing a “pedestrian intent prediction platform”, is one of the few known startups working on incorporating intentions and behavioural sciences. There may be others still in stealth mode. By solving one of the most pressing challenges in the deployment of safe autonomous vehicles, Perceptive Automata is poised to emerge as one of the key players in the landscape.
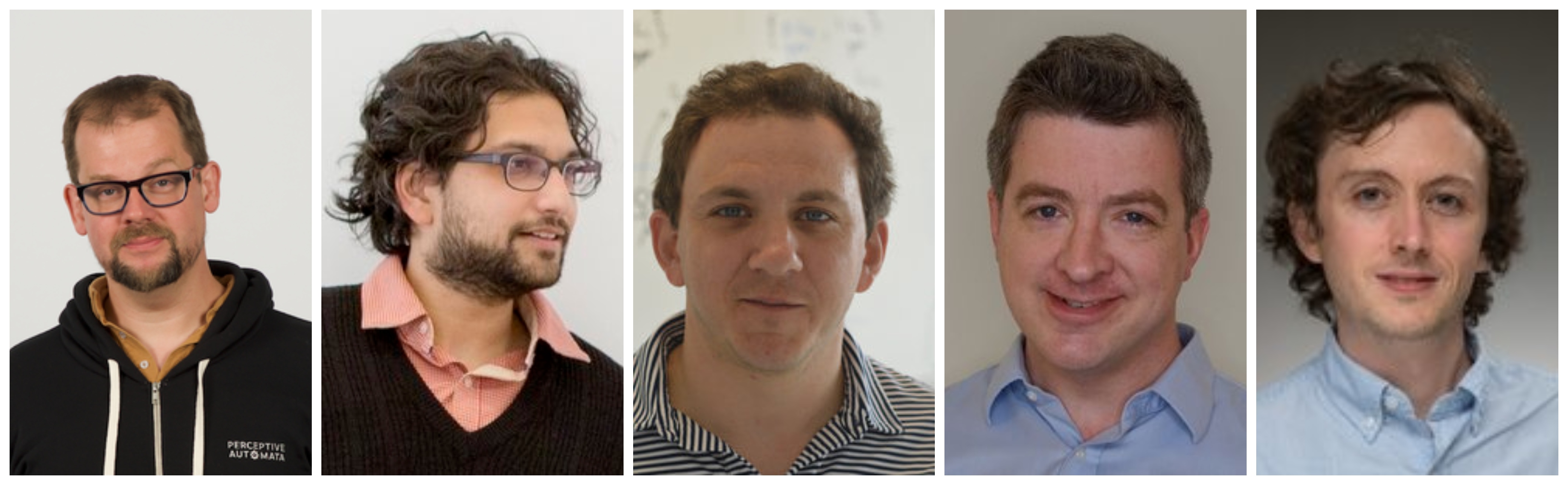
The co-founders (R-L) Dr. Sam Anthony (CTO), Sid Misra (CEO) Avery Faller, Dr. David Cox and Dr. Walter Scheirer (PC: Perceptive Automata)
Subscribe to our newsletter